“All models are wrong, but some of them are useful”, said George Box, a British statistician, almost 50 years ago. In reality, many agricultural operations rely on the results of various models — from the weather forecast to yield prediction.
Setting up right yield goals and forecasting yield during the growing season is the Holy Grail of precision agriculture. Both USDA and Statistics Canada, as well as some private companies, forecast grain yield during the growing period at the country scale, using remote sensing data, and they do it quite accurately. At the same time, yield forecast at the field or site-specific level has always been a challenge; the results of such forecast can be strongly affected by the influence of crop varieties, soil moisture and fertility levels, and many other factors. Using the same grain yield forecast algorithms for site-specific yield prediction can easily result in 30 to 50 per cent error. Therefore, to achieve better accuracy, yield forecast at the field or management zone level requires additional ground truthing information.
A vegetation index map, e.g. Normalized Difference Vegetation Index (NDVI), at the peak of the growing season, in most cases, is strongly correlated with the grain yield. Previously, we have shared the results of such forecast, using high-resolution drone imagery and field scouting. However, to convert NDVI values into the grain yield information, we need the results of ground truthing. The best criterion of the site-specific yield forecast is to compare predicted results with the actual yield map collected by a calibrated yield monitoring system.
To forecast grain yield in spring wheat, we used a multispectral satellite image from Sentinel 2A collected on July 25, 2021. An NDVI dataset was created from that satellite image and clipped to the field boundary (Smart Farm, Field 18).
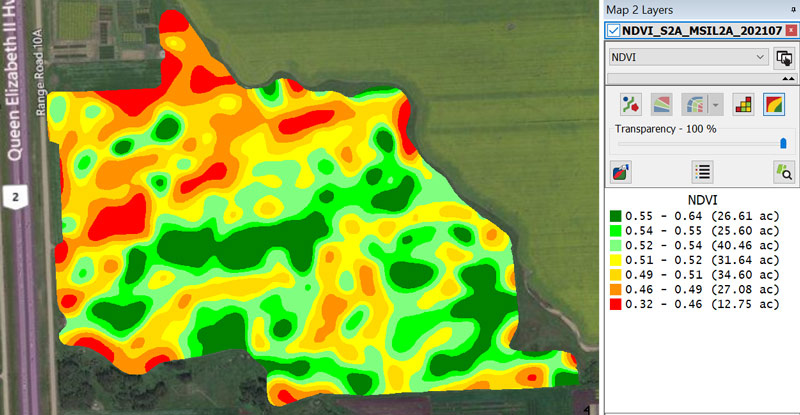
Fig. 1. NDVI map, July 25, 2021. Olds College Smart Farm, Field 18.
To forecast grain yield, our Digital Ag team used this NDVI map for navigation in the field and collection of the ground truthing data.